Demonstrate ECM Extractors#
The ECM extractors assemble the required components for Equivalent Circuit model using reference performance test data.
[1]:
%matplotlib inline
from matplotlib import pyplot as plt
from battdat.data import BatteryDataset
import numpy as np
Load an Example Dataset#
We use an example RPT cycle from the CAMP 2023 dataset to demonstrate parameter extraction
[2]:
data = BatteryDataset.from_hdf('files/example-camp-rpt.h5')
C:\Users\lward\AppData\Local\miniconda3\envs\moirae\lib\site-packages\battdat\data.py:57: UserWarning: Metadata was created in a different version of battdat. supplied=0.4.0, current=0.4.1.
warnings.warn(f'Metadata was created in a different version of battdat. supplied={supplied_version}, current={__version__}.')
[3]:
data.tables['raw_data']
[3]:
cycle_number | file_number | test_time | state | current | voltage | step_index | method | substep_index | cycle_capacity | cycle_energy | |
---|---|---|---|---|---|---|---|---|---|---|---|
0 | 1 | 0 | 120.042 | b'charging' | 0.260548 | 3.450065 | 0 | b'constant_current' | 0 | 0.000000 | 0.000000 |
1 | 1 | 0 | 139.182 | b'charging' | 0.260090 | 3.470207 | 0 | b'constant_current' | 0 | 0.001384 | 0.004789 |
2 | 1 | 0 | 439.182 | b'charging' | 0.260014 | 3.487297 | 0 | b'constant_current' | 0 | 0.023055 | 0.080177 |
3 | 1 | 0 | 739.182 | b'charging' | 0.259937 | 3.493553 | 0 | b'constant_current' | 0 | 0.044720 | 0.155796 |
4 | 1 | 0 | 1039.182 | b'charging' | 0.260166 | 3.499809 | 0 | b'constant_current' | 0 | 0.066391 | 0.231572 |
... | ... | ... | ... | ... | ... | ... | ... | ... | ... | ... | ... |
180 | 1 | 0 | 41767.320 | b'hold' | 0.000000 | 3.272145 | 3 | b'rest' | 8 | -0.027665 | 0.011427 |
181 | 1 | 0 | 41777.322 | b'hold' | 0.000000 | 3.276875 | 3 | b'rest' | 8 | -0.027665 | 0.011427 |
182 | 1 | 0 | 41787.318 | b'hold' | 0.000000 | 3.280995 | 3 | b'rest' | 8 | -0.027665 | 0.011427 |
183 | 1 | 0 | 41797.320 | b'hold' | 0.000000 | 3.284504 | 3 | b'rest' | 8 | -0.027665 | 0.011427 |
184 | 1 | 0 | 41807.310 | b'hold' | 0.000000 | 3.287556 | 3 | b'rest' | 8 | -0.027665 | 0.011427 |
185 rows × 11 columns
The dataset contain a single slow cycle that samples the entire capacity of the cell.
[4]:
fig, axs = plt.subplots(2, 1, figsize=(3.5, 3.), sharex=True)
raw_data = data.tables['raw_data']
time = (raw_data['test_time'] - raw_data['test_time'].min()) / 3600
axs[0].plot(time, raw_data['voltage'])
axs[0].set_ylabel('Voltage (V)')
axs[1].plot(time, raw_data['current'])
axs[1].set_ylabel('Current (A)')
axs[1].set_xlabel('Time (hr)')
[4]:
Text(0.5, 0, 'Time (hr)')
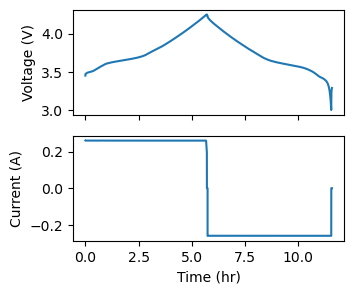
Determining Capacity#
The MaxCapacityExtractor
determines capacity by integrating current over time then measuring difference between the maximum and minimum change in charge state.
Note: Integration is actually implemented in battdat.
[5]:
from moirae.extractors.ecm import MaxCapacityExtractor
[6]:
cap = MaxCapacityExtractor().extract(data)
cap
[6]:
MaxTheoreticalCapacity(updatable=set(), base_values=array([[1.48220808]]))
This should match up with the measured change in charge
[7]:
fig, ax = plt.subplots(figsize=(3.5, 2.))
ax.plot(time, raw_data['cycle_capacity'])
ax.set_xlim(ax.get_xlim())
ax.set_ylabel('Capacity Change (A-hr)')
ax.set_xlabel('Time (hr)')
min_cap, max_cap = raw_data['cycle_capacity'].min(), raw_data['cycle_capacity'].max()
ax.fill_between(ax.get_xlim(), min_cap, max_cap, alpha=0.5, color='red', edgecolor='none')
[7]:
<matplotlib.collections.PolyCollection at 0x2274cab1f90>
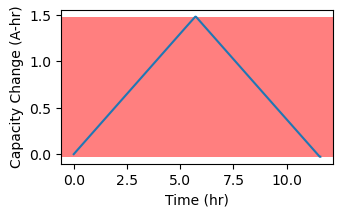
The width of the colored span is the estimated capacity and it spans from the lowest capacity (~11hr) and the highest (~6hr)
Open-Circuit Voltage Estimation#
The OCVExtractor
determines the Open Circuit Voltage by fitting a spline to voltage as a function of state of charge.
The first step is to compute the SOC from the capacity change during the cycle and the total capacity estimated by MaxCapacityExtractor
. As the actual state of charge cannot be directly measured, we assume the lowest capacity change is an SOC of 0.
[8]:
raw_data['soc'] = (raw_data['cycle_capacity'] - raw_data['cycle_capacity'].min()) / cap.base_values.item()
[9]:
fig, ax = plt.subplots(figsize=(3.5, 2.))
ax.plot(raw_data['soc'], raw_data['voltage'])
ax.set_ylabel('Voltage (V)')
ax.set_xlabel('SOC')
[9]:
Text(0.5, 0, 'SOC')
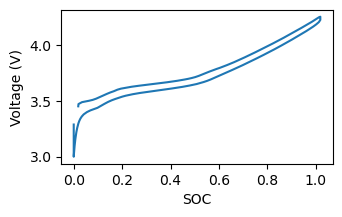
The voltage during charge and discharge are different. Moirae accounts for this by weighing points with lower current more strongly and fitting a smoothing spline.
Note: Make the spline fit data more closely by increating the number of SOC points
[10]:
from moirae.extractors.ecm import OCVExtractor
ocv = OCVExtractor(capacity=cap).extract(data)
[11]:
fig, ax = plt.subplots(figsize=(3.5, 2.))
ax.plot(raw_data['soc'], raw_data['voltage'], label='Data')
soc = np.linspace(0, 1, 64)
fit = ocv(soc)
ax.plot(soc, fit[0, 0, :], 'r--', label='OCV')
ax.set_ylabel('Voltage (V)')
ax.set_xlabel('SOC')
ax.legend()
[11]:
<matplotlib.legend.Legend at 0x2274db7dc00>
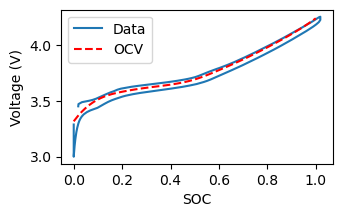
This yields a generally-good representation of the OCV.
[ ]: